Ecological monitoring is a key part of any conservation work: if you don’t know what’s out there, you can’t protect it. But that’s easier said than done: using traditional survey methods, this work is costly, logistically challenging, slow and limited in its geographic scale. In the last couple of decades, two approaches have emerged to speed up the process of collecting and processing data. The first is citizen science, which recruits non-expert volunteers to record data in the field, as well as help make sense of it. The second is AI, which doesn’t help collect raw data, but with the right training, can learn to process and label data as accurately or more accurately than humans, in a fraction of the time. Both methods are already helping to scale conservation efforts — but they’re not interchangeable. In this post I’ll navigate some of the trade-offs they introduce, and how those depend on the use case at hand.
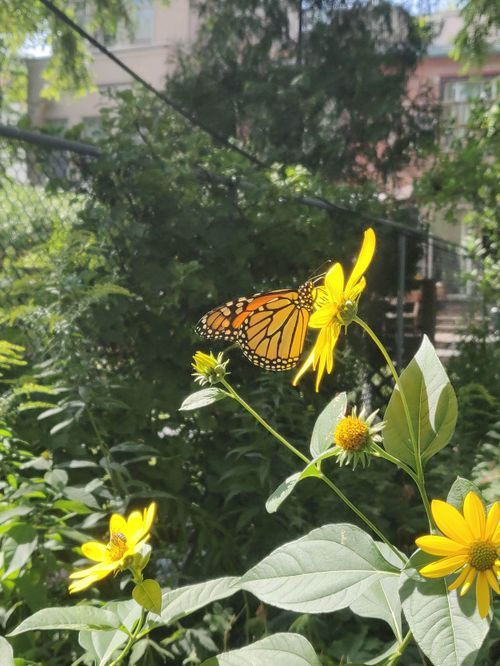
One review of projects using citizen science and AI in ecology highlights the potential for complementarity between the two approaches, since citizen science provides data at a geographic and temporal scale that traditional monitoring techniques have trouble achieving. Because of this, citizen science initiatives have excelled in monitoring poleward shifts in animal species ranging from butterflies to marine fish. Citizen science projects also build valuable public engagement in conservation issues. However, since citizen science relies on volunteers, it is most effective for accessible locations and charismatic target species, and may lose traction as the project ages.
A more serious limitation of citizen science is its potential for inaccuracy. A quantitative review of hundreds of published citizen science studies found that while only 51-62% of published comparisons resulted in accurate citizen science data, 73% of abstracts described the accuracy of the data in positive terms. This suggests that the accuracy of citizen science data is generally over-reported. What does this mean for integration with AI?
There’s a common view of AI applications that if only we could get enough data, the problem would be all but solved. While there are lots of cases where data quantity is the limiting factor, there are as many more where it’s not. A less well-known trend in AI is that data quality can make or break the success of algorithms. Andrew Ng, a leading figure in AI, recently wrote an article underlining the pivotal role of data quality over quantity, especially in smaller-scale applications (this includes practically all ecological applications).
Even when quantity is no object, problems with quality can prove detrimental. The worldwide effort to use AI to aid in COVID-19 diagnosis, which is now widely agreed to have been a failure, is a cautionary tale here. At the beginning of the pandemic, numerous independent groups of AI scientists dropped what they were doing and put themselves to the task of diagnosing and triaging patients using x-ray and CT scan imagery. As the virus spread, so did large datasets of labelled medical imagery. But the doctors submitting the imagery — understandably — had other things on their minds besides ensuring data uniformity. Doctors are professional doctors, but when it comes to annotating data for machine learning, they’re citizen scientists. A number of problems emerged with the data. Combining datasets from multiple sources sometimes led to duplicates, cross-contaminating the testing and training datasets and artificially inflating success metrics; some algorithms learned that horizontally-oriented x-rays were more likely to be seriously ill than vertically-oriented ones (if you’re seriously ill, you’re likely lying down); and so on. The result was that, despite hard work and good intentions on all fronts, no reliable diagnostic tool ever came out of these efforts. Big data abounded, but good data did not.
So the question is: is the bottleneck for marine mammal detection big data, or good data? The field is witnessing an explosion of new, increasingly affordable, data gathering techniques. We can even see whales from space! This leaves data processing and analysis — not data collection — as the tightest bottleneck. That’s not to say citizen science can’t play a role down the road, but Whale Seeker believes that the first step to a reliable and scalable monitoring solution will have to depend on an interplay between expert biologists and AI.